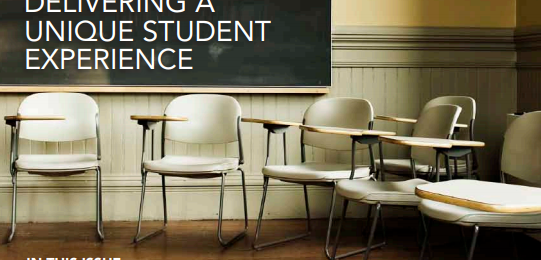
In this report:
- Where Current Retention Efforts Fall Short
- Starting with Fit: Defining and Delivering the Unique Student Experience
- Designing the Student Experience: Building Bridges across Student and Academic Affairs
- Delivering on the Promise: Removing Barriers to Student Success
- Identifying and Intervening with At-Risk Students
April 2011. Large organizations in any industry often suffer from a misalignment of people, policies, and practice. Colleges and universities are no different. And the issue of student retention is a prime example of when good intentions, money, and technology aren’t enough to overcome policies, structures, and incentives that work at odds with one another.
Amid the increased public pressure on completion rates, many colleges are pursuing one-off programs whose impact is sometimes difficult to measure. Less focus has been paid to the need for a broader conversation of organizational alignment as a means of tackling the root causes which contribute to student attrition, increased costs, and external demands for accountability.
The first step in promoting student success is to define the specific educational experience and value an institution offers to students — and then align recruitment strategy, organizational structures, student support initiatives, academic policies, and incentives to support that experience and ensure that the institution delivers on its promise.
That’s why we’ve convened a cadre of experts in higher education policy, research, and practice to present an integrated, strategic view of the challenges and opportunities for addressing student success. We hope their insights and advice will be useful to you.