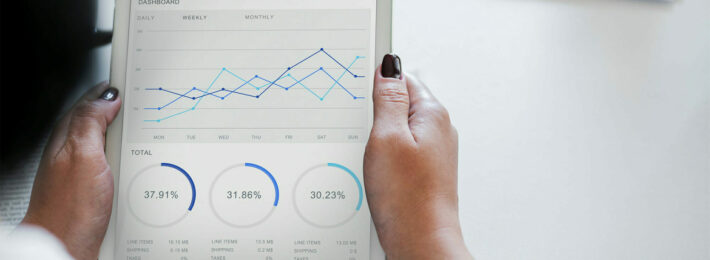
Are we looking at student data in ways that help us improve our efforts? What holds us back from measuring and improving student success?
What holds us back from measuring student success and implementing measures to improve it? How should every institution be looking at its student data differently? These are pressing questions to answer. Let me share a few of the things I’ll be talking about at our upcoming conference Building Your Data Strategy to Improve Student Success Programs and Interventions. I want to share four steps all institutions need to take—and three examples of who is already doing this.
4 Things to Do
We need to have institution-wide strategies to improve student success programs and interventions. And for these strategies to work, we have to define the strategic needs and priorities that you we expect from analytics and align investments in financial and human resources to build the analytics required to improve student success. (For more on what that might look like, see Ronald Yanowski’s article “The Analytics Landscape in Higher Education.”
Here are four things every institution needs to do:
1. Focus on the right questions and adopt a comprehensive analytics strategy to address them.
Often in higher education, data are collected but rarely used or never used at all. In fact, while some institutions are using institutional analytics to measure operational monitoring, fewer institutions are using learning analytics or using data to address student success. We need to be calculating which students are at-risk, what programs are used for interventions, what impact the programs have in the short-term and long-term is critical if sustainable progress is to be made in student success.
And we need a comprehensive analytics strategy. We need to view data in terms of what helps institutions make better decisions more often about student success. What data will help campuses do a better job?
2. Establish a dedicated—and holistic—team.
For institutions to focus on outcomes, actions and impact they must develop a student success team that collaboration across siloed divisions and service areas. Currently, there are isolated analytics capabilities where resources are fragmented or non-existent. This is complicated work. Institutions need to have people who can bridge or translate data science with on-the-ground operations. Change agents can serve as culture catalysts and translators. The following steps are important:
- Need clearly defined analytics roles both in the present and for the future.
- Don’t isolate the analytics capabilities away from the front lines. Buy in and use requires the action providers to be in the data decision conversations.
- Too often institutions bolt analytics to aging legacy systems. Modern architecture and up-to-date data platforms are critical for long-term success.
- Clear focus is needed to identify ethical, social and regulatory implications of analytics initiatives.
- It takes a high functioning, cross-expertise team. Currently the team should include IT, IR, Student Services, Academic Support, Budget, and Communications. More emphasis is needed on developing analysts, and Student Success Scientists.
3. Conduct a total data strategy audit.
This audit that includes what data is available for which students, in what context, and for what purpose:
- Determine who is responsible for student data and clarify roles and responsibilities. Determine what is being done in student interventions and what is working.
- Audit the data infrastructure for capacity to support and sustain growth.
- Review and address human and fiscal needs to build and sustain an integrated student data culture and strategy.
4. Develop a Student Success Mindset.
We need to do myth busting in order to move forward. As EDUCAUSE recently stated in The Human Genome Project, analytics alone can’t improve student success and retention. It is time to debunk some of the misconceptions and myths that exist on campuses, such as:
- Myth: These are initiatives that you start and finish.
Instead they are social experiments that run for decades. Analytics expert Don Norris calls it an “expedition.” - Myth: Student success is one area’s responsibility.
Instead, the complexities of these problems require a variety of people’s input and efforts to progress. - Myth: Student success is the student’s problem.
Institutions must change the mindset and take a personal approach to helping individual students succeed. Institutions must work to engineer failure out of the system.
3 Examples of Who Is Doing Student Data Analytics Well
Austin Community College
Austin Community College determined that they would re-imagine student success across the campus. They realized that to make improved student success a reality, they needed to focus on the total experience of the student. Every unit needed to claim student success as its goal. This required strong leadership to communicate that this was a number one priority. It also required a major coordination of student services that had previously been fragmented and siloed. It required changing the processes that students experienced so as to improve their access to the services and their success.
The college determined that the right data, the right people, and the right tools were essential for a total re-imagined approach to student success.
What ACC has achieved:
- Increased dual credit and early college high school participation.
- Increased overall enrollment and diversity.
- Increased the use of tools to increase use of predictive measures to reduce at risk behavior
- Increased career and guided pathways
- Increased adult education
- Increased persistence
- Began closing the gaps of successful course completion:
Students who start at ACC are also persisting at higher rates. Successful course completions (grades of A, B, C) in all courses, including developmental education, in the first semester for FTIC (First Time in College) students increased 12 percentage points (59 percent in 2009 to 71 percent in 2016). Here is more information.
University of Arizona
The University of Arizona focused on gaining a “single source of truth” in data. This was critical to create the strong data foundation required to move student success planning. They examined the student lifecycle from recruitment to completion to career assessing—where students gained momentum and success and where they ran into barriers to success. Using this data, the University of Arizona worked to optimize financial aid to maximize yield, diversity, and quality. They expanded student success programming, student engagement opportunities, and trained people in working with at-risk students, in improving advising for success, and in using data to improve decision making. This included building advisor dashboards that allowed advisors to identify students with an array of shared risk factors.
What the University of Arizona has achieved:
- 3% lift in FTFT retention
- 6% lift in 6-year graduation
- Refined retention program parameters
- Colleges doing outreach campaigns 2x/term
- Issue-specific campaigns throughout the year
- Midterm grades for >90% of freshmen
- More Supplemental Instruction
- Funding for enrollment caps in writing classes
St. Cloud State University
St. Cloud State University has had a history of building data capacity. They have systematically developed the build-out of a data/analytics capacities and have focused on improved coordination across offices and intervention opportunities. SCSU has focused on mid-range performance on student success metrics including retention, credit-taking behavior, and engagement.
While the campus had already developed big metrics, there was still a critical need for actionable information. They focused on engaging stakeholders in discussion of the intersection of data, technology, and communication; expanded their data capabilities linked with action; and worked to monitor progress and improvements. This has included expanded adoption of analytics platforms. They are using the DELTA maturity model, which provides a strategic framework for assessing data, the enterprise, leadership, targets, and analysts; the model is adapted from the Five Stages of Analytics Maturity developed by Tom Davenport and Jeanne Harris in their book, Competing on Analytics: The New Science of Winning, and the DELTA Model developed in 2010 by Tom Davenport, Jeanne Harris, and Bob Morison in their book, Analytics at Work: Smarter Decisions, Better Results.
What SCSU has accomplished:
- Fall-Spring Retention: +3.2%
- Average credit-taking behavior: +0.77
- Closed the registration gap
- Expanded problem-specific interventions:
- Adopted research and engagement based on a belonging mindset
- Improved the Student Success Center
- Developed pop-up advising opportunities
- Developed targeted outreach