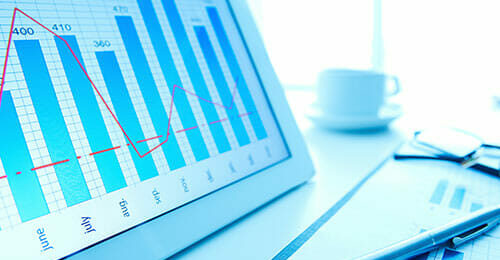
Included in This Report:
- Reviewing Your Data: What You Might Find
- Data-Informed Recruitment and Enrollment
- A Data-Informed Approach to Student Retention
- Mining Your Data: From Students to Alumni
October 2012. Given increasing competition, shifts in student enrollment, and reduced resource levels, it’s critical that colleges and universities recruit and retain the students who are most likely to succeed at their institutions.
By reviewing data on current and past students and alumni, and engaging in predictive modeling, you can identify not only the factors that impede desired outcomes such as yield, student retention, and alumni engagement and giving rates, but also the positive factors that contribute to those outcomes.
In this edition, we have turned to institutional researchers, enrollment managers, and advancement professionals to highlight examples of predictive indicators and data-informed tactics for enrolling and supporting the right students and helping them transition into engaged, committed alumni. We hope their advice will be helpful to you.