Using Retention Metrics to Support At-Risk Online Students
Last Updated
September 23, 2013
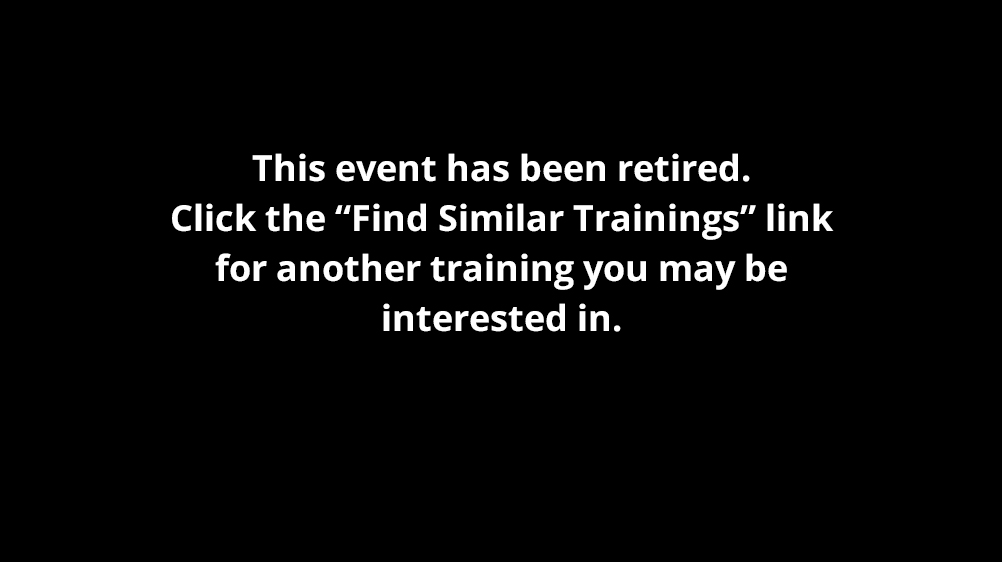
Using Retention Metrics to Support At-Risk Online Students
Table of Contents
Overview
The human and technological resources needed to ensure student success online are expensive to acquire and difficult to maintain. Consequently, institutions must make data-driven decisions about where to invest their resources. However, the data needed to inform such choices can be hard to isolate and are subject to misinterpretation given the complexities and constantly changing characteristics of online education.
Examine how you can use metrics to better support retention efforts and improve the success rates of your online students. This webcast will address the use of data for modeling and prediction, and evaluating the effectiveness of interventions, including:
- Data available to your institution
- Mechanisms for obtaining data
- Conclusions that can and cannot be drawn from data about your retention and success initiatives
- Identifying at-risk students through analysis of predictive behaviors
- Using interventions to help reduce the risks
- Monitoring the interventions for effectiveness
Who should attend?
If you want to use data and analytics to better inform and improve your retention efforts, and learn some intervention practices, then this program is for you. Fictitious student profiles will be used throughout the webcast as a way to emphasize the points. Academic leaders, online program managers, academic advisors, and retention administrators will leave this webcast better able to integrate data and analytics into your retention practices.
Note: this session does not cover the fundamentals of conducting statistical analysis.
Agenda
- Factors leading to attrition
- Levels of measurement & data sets
- Using data for modeling and prediction
- Indicators of risk
- Student behaviors
- Academic performance
- Availability of interventions and pathways (for referring students to them)
- Strengths and weaknesses of predictive modeling
- Indicators of risk
- Using data for evaluating effectiveness of interventions
- Census (individual student) data
- Sampling
- Best practices in intervention
- Takeaways
Tagged In
Why is this event retired?
At AI we want to always ensure that the best and most current trainings are available to members, and we regularly review our trainings to ensure that is the case.