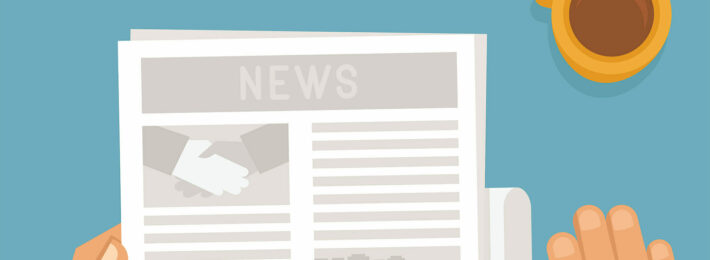
Most alumni relations and annual giving operations have limited intelligence about who will be a strong reunion volunteer, annual giver, or alumni travel prospect—if the person has not previously participated in any of those activities. But rather than pulling a random database query and then reaching out at random to the contents of the entire resulting list, applying predictive analytics can help provide a more targeted allocation of your resources and more targeted messaging.
Why Predictive Modeling is Critical
Many organizations seeking to improve operating performance are turning to predictive analytics and predictive modeling to either increase revenues, decrease costs, or both. The size of our alumni population and database at Penn make focusing resources critical to fundraising success. The objectives of our early pilot projects in predictive modeling have been to reduce fundraising-related marketing costs and/or increase the dollars raised, or improve business performance in some other way.
Here are examples of how our recent analytics efforts at Penn have informed critical resourcing decisions:
- We have been able to focus on those prospects most likely to be donors. Targeting the 40% mostly likely to donate can save mailing costs, phone solicitation costs and even development officer time and travel expenses.
- We have been able to target the marketing for our Alumni Travel programs. Here, predictive modeling focuses the direct mail campaigns on those most likely to take a Penn Alumni trip, saving mailing costs and/or increasing alumni response rates.
- Recruiting reunion planning and gift committee members each year starts with an invitation to 25,000+ alumni to a Reunion Planning Leadership Conference. Because this “call” for volunteers may not yield a sufficient number of volunteers, predictive modeling has been used to identify another 300–500 of the best potential volunteers for personal contact by alumni relations and/or reunion annual giving staff.
The Steps for Effective Predictive Modeling
The steps in the predictive modeling process are:
- Identify the business need
- Define the problem
- Assemble the data
- Condition the data
- Build the model
- Revise and test
- Implement the results
Identification of the business need and a proper problem definition are critical for a successful project. In most data warehouse systems, the data is encoded in ways that are not immediately useful for predictive modeling. Basically, the data as it exists in the data warehouse will require transformation and conditioning to be useful for predictive modeling. Assembling and conditioning the data can easily account for 90% of the total project effort.
Building the model once the data has been prepared is relatively easy using available software tools. Revising and testing is also relatively easy compared to the work of conditioning the data. Implementation will depend on how the organization will use the model results. Some models are used by only a few people, while others will be used across the entire organization and will need to be available in the operations database.
Achieving This at Low Cost, with Few Staff
SOFTWARE. While more sophisticated modeling software has been used for the applications in this report, most of the predictive models we have been using can also be run using the data analysis tools provided in Microsoft Excel.
STAFF. The projects we referred to in this article were the collaborative work of only two analytics professionals—both of whom had other analytics project responsibilities.
And you can get started even with fewer resources.
Your institution may have students and faculty with the background and expertise to use the techniques described here. A partnership between fundraising professionals and academics could be an ideal low-cost approach to get started in predictive modeling.
Review What We’ve Learned
Using predictive analytics, the University of Pennsylvania has found a series of model variables that identify strong prospects. In our 2013 monograph Using Predictive Modeling to Guide Resource Allocation in Annual Giving and Alumni Relations, we walk you through the independent variables that Penn found to be statistically significant and helpful in guiding resourcing decisions. With these variables in hand, you’ll be able to more effectively search your own database to find the best prospects for various alumni relations and annual giving functions.
In our report, you can read about using predictive modeling to target:
- Alumni for reunion-year annual giving
- Alumni for giving in a non-reunion year—especially looking for prospects among lapsed and never givers
- Institutional “friends” for annual giving
- Prospective alumni reunion volunteers
- Alumni who are most interested in alumni tours
If you already have an advanced knowledge of predictive analytics, our report offers you insights on variable definition and selection, model structure, data conditioning, and assessment of model “validity” and performance.
If you have little or no prior background in predictive modeling, our report offers an understanding of how one uses predictive modeling, the data requirements, the results you can obtain, and the potential impact for your organization.
If this article has been useful in challenging or inspiring your thinking, we hope you will consider ordering our 100-page monograph for a thorough primer on predictive modeling for annual giving and alumni relations. You can learn more here.
Academic Impressions offers a full suite of conferences, workshops, and online trainings for advancement professionals, covering every aspect of the advancement operation and bringing you the innovations you need to meet this year’s challenges. We encourage you to take a look at our related page listing in-depth events and online trainings for advancement professionals.